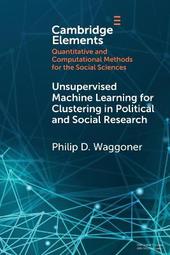
|
Unsupervised Machine Learning for Clustering in Political and Social Research
Paperback / softback
Main Details
Description
In the age of data-driven problem-solving, applying sophisticated computational tools for explaining substantive phenomena is a valuable skill. Yet, application of methods assumes an understanding of the data, structure, and patterns that influence the broader research program. This Element offers researchers and teachers an introduction to clustering, which is a prominent class of unsupervised machine learning for exploring and understanding latent, non-random structure in data. A suite of widely used clustering techniques is covered in this Element, in addition to R code and real data to facilitate interaction with the concepts. Upon setting the stage for clustering, the following algorithms are detailed: agglomerative hierarchical clustering, k-means clustering, Gaussian mixture models, and at a higher-level, fuzzy C-means clustering, DBSCAN, and partitioning around medoids (k-medoids) clustering.
|